Predictive analytics, powered by data and artificial intelligence (AI), has become a cornerstone in modern healthcare. The application of predictive analytics and predictive modeling has allowed healthcare organizations to optimize operations, enhance patient care, and reduce costs significantly.
How Predictive Analytics Works
Predictive analytics requires structured historical data, which is fed into mathematical models to identify patterns and trends. These models, powered by predictive algorithms, analyze the current data and predict future outcomes. In healthcare, this process enables organizations to address critical issues preemptively, potentially saving lives and improving operational efficiency.
Key steps involved in predictive analytics include:
- Identifying a specific problem to solve.
- Preparing and cleaning data for analysis.
- Developing and testing models.
- Deploying predictive algorithms to generate actionable insights.
Data Sources in Healthcare
Effective predictive analytics requires robust data inputs. These include:
- Structured Data: Patient records, bed availability, and ICU occupancy rates.
- Unstructured Data: Public case studies and external reports that offer broader insights.
- Global and Regional Data: Information from centralized bodies (like NHS England) to local healthcare providers ensures comprehensive modeling.
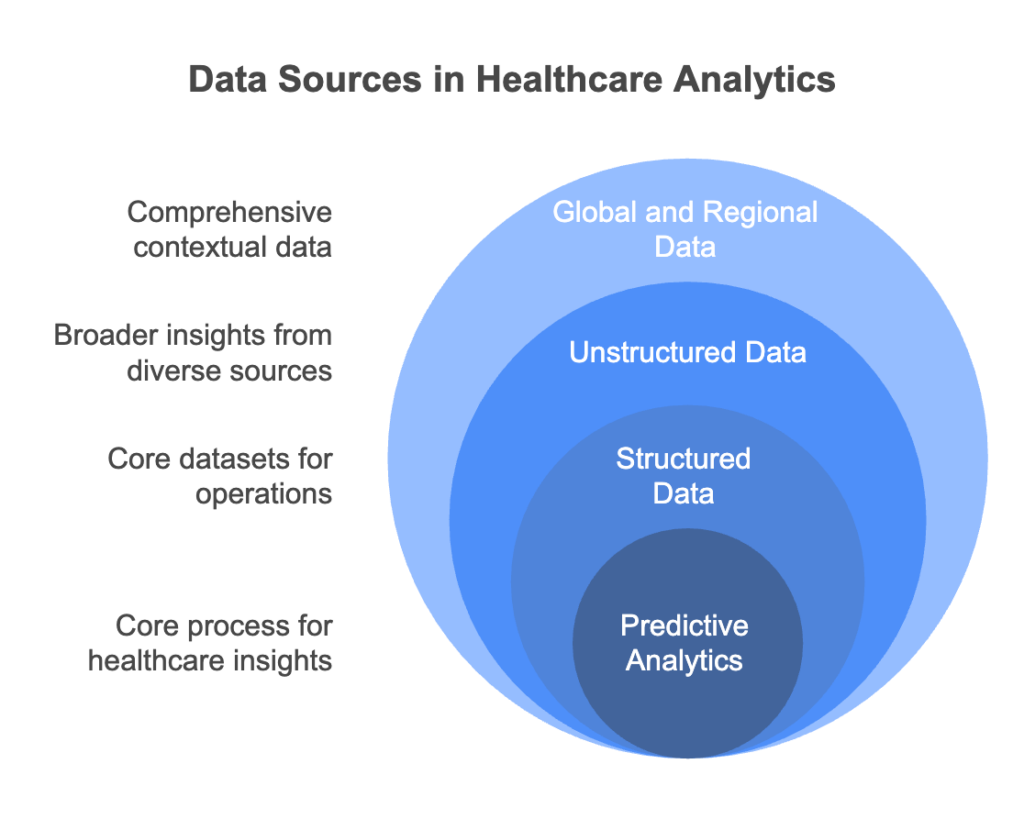
How Predictive Analytics is Implemented
Implementing predictive analytics in healthcare involves several key steps, including data acquisition, model development, and integration into clinical workflows. However, achieving scalable and effective solutions often requires specialized expertise in cloud platforms and data engineering.
At Gart Solutions, we specialize in creating and deploying scalable cloud-based solutions tailored to the unique needs of healthcare organizations. Our expertise in platform engineering ensures that predictive analytics models are integrated seamlessly into your existing infrastructure, enabling real-time insights without compromising data security or compliance.
*Implementing predictive analytics in healthcare involves navigating ethical concerns, particularly regarding data privacy and algorithmic biases. Regulatory frameworks must evolve to address these issues, ensuring the technology’s responsible and equitable use.
AI Models and Technologies
AI applications in healthcare rely on advanced models and technologies:
- Machine Learning: Algorithms predict patient trends and optimize workflows.
- SIR Models: These epidemiological models assess infection rates and healthcare demands.
- Cloud Solutions: Hospitals adopt cloud-based platforms for scalability and remote access to predictive tools.
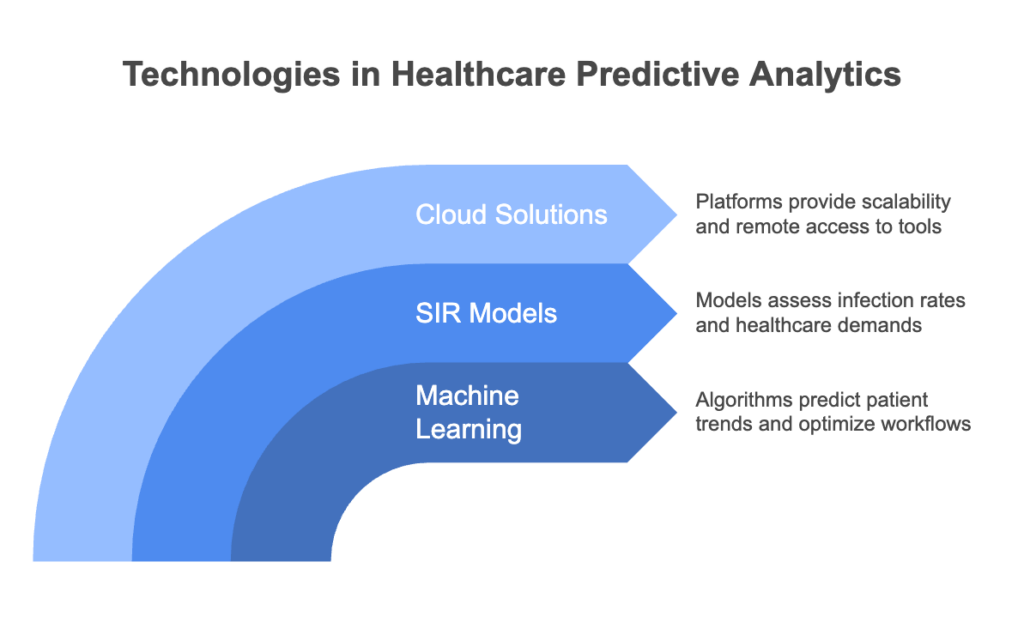
Predictive analytics has found diverse applications within the healthcare sector:
Risk Assessment and Disease Prediction
Predictive models aid in identifying patients at risk of developing chronic conditions such as diabetes, heart disease, or Parkinson’s disease. For example, machine learning algorithms analyze extensive medical data to detect early warning signs, allowing for timely interventions.
Patient Care & Personalized Medicine
Hospitals can leverage predictive models to anticipate patient outcomes, especially in high-stakes environments like intensive care and emergency rooms. Alerts generated through these models can lead to timely interventions, reducing mortality and complications.
By analyzing individual genetic data, clinical history, and lifestyle factors, predictive analytics supports the development of customized treatment plans. This tailored approach improves therapy effectiveness and patient satisfaction.
Readmission Prediction
By analyzing data from high-risk populations, healthcare providers can forecast the likelihood of patient readmissions and implement preventative measures, improving care quality and reducing penalties associated with readmission rates.
Financial Insights
Predictive models allow institutions to calculate potential savings or losses and optimize resource allocation. This can lead to significant cost reductions while maintaining or improving service quality.
Preventive Medicine
Advanced modeling, including genetic data analysis, facilitates early diagnosis and treatment planning, preventing disease progression. This proactive approach reduces unnecessary healthcare expenditures and enhances patient outcomes.
Resource Allocation and Population Health
Healthcare systems utilize predictive models to stratify patient populations, prioritize care for high-risk groups, and allocate resources effectively. This approach optimizes operations and reduces costs.
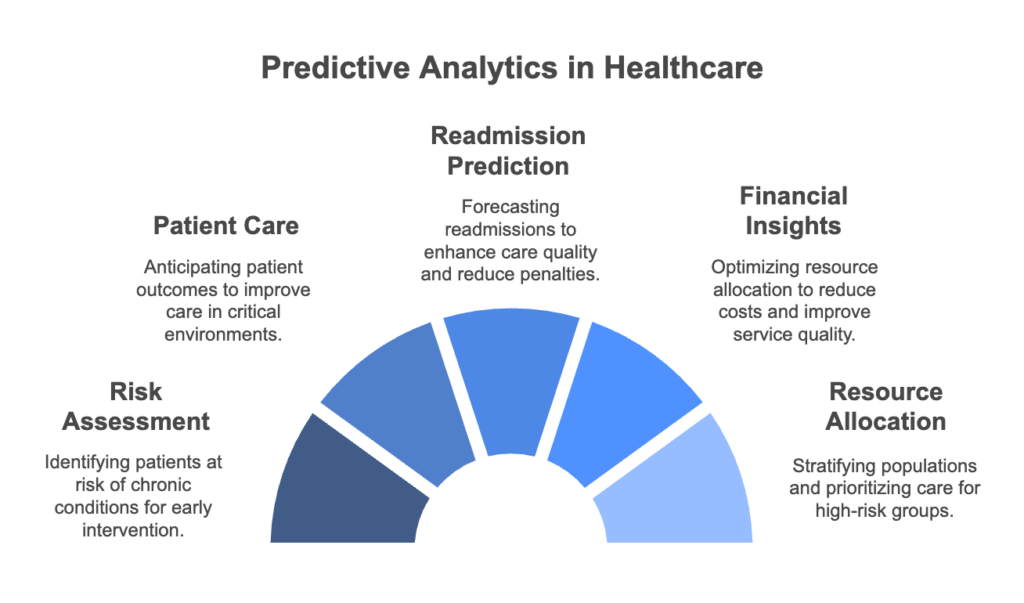
Gart Solutions has worked with healthcare providers to design cloud architectures that support these applications. From enabling real-time data processing to ensuring HIPAA-compliant storage solutions, we provide the technological foundation for innovative healthcare analytics.
The Role of Predictive Modeling
Predictive modeling employs statistical techniques to forecast future behaviors and outcomes. It has grown steadily within healthcare, helping providers:
- Manage complex patient populations effectively.
- Implement value-based care strategies.
- Use decision trees for transparent, explainable predictions, crucial for auditing and compliance purposes.
For instance, decision tree models classify new cases based on historical data and enable healthcare organizations to refine their approach to patient care and financial planning.
Predictive analytics offers numerous advantages:
- Improved Operational Efficiency: Predictive tools help streamline scheduling, resource utilization, and other organizational processes.
- Cost Reduction: Predictive insights can lead to a 20-30% reduction in overall healthcare spending by identifying inefficiencies.
- Enhanced Patient Experience: Personalized care models based on predictive analytics improve patient engagement and satisfaction.
Methodology for Developing Predictive Models
1. Data Acquisition and Integration:
Data from electronic health records (EHRs) provides comprehensive insights into patients’ health histories. This step involves collecting and integrating data such as diagnoses, medications, and lab results.
2. Feature Engineering and Selection:
Exploratory data analysis (EDA) identifies significant variables for prediction, ensuring models are both clinically relevant and accurate.
3. Model Development and Training:
Supervised learning algorithms, such as decision trees and neural networks, are trained on labeled datasets to predict outcomes. Fine-tuning through hyperparameter optimization ensures robust performance.
4. Deployment and Integration:
Scalable, user-friendly interfaces present predictions and insights to healthcare professionals through interactive dashboards. Usability testing ensures the tools are intuitive and practical.
5. Continuous Monitoring and Improvement:
Predictive models require regular updates to maintain accuracy as new data becomes available. Automated monitoring systems detect and address performance issues, ensuring models adapt to changing healthcare needs.
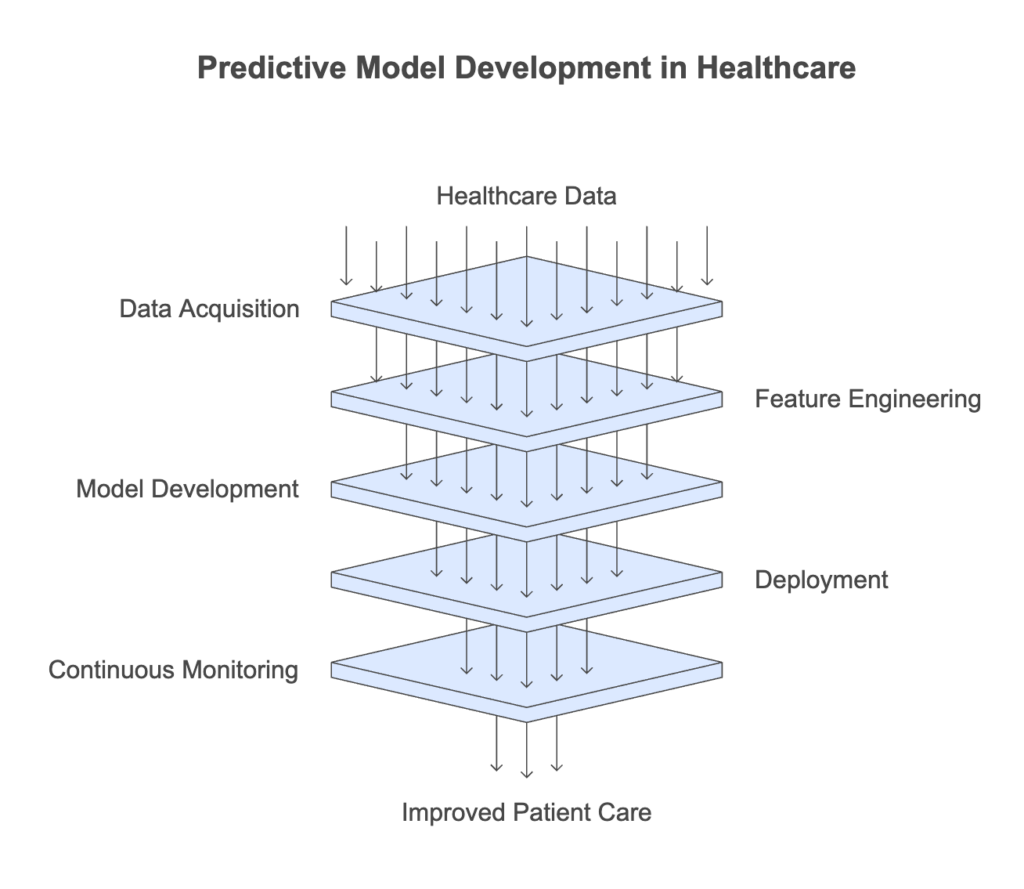
Case Studies in Disease Prediction
Predictive analytics has demonstrated remarkable success in improving disease detection and management. The following case studies highlight its application across various chronic conditions:
Parkinson’s Disease:
A 2018 study titled “Parkinson Disease Prediction Using Machine Learning Algorithm“ showcased the power of predictive models in identifying early indicators of Parkinson’s disease. The study employed machine learning to sift through clinical data, identifying key biomarkers and patterns associated with the disease. These findings enable healthcare providers to detect the condition earlier, allowing for timely intervention and better management of this progressive neurological disorder.
Heart Disease:
Research in 2019, titled “Effective Heart Disease Prediction Using Hybrid Machine Learning Techniques,” explored a hybrid approach combining multiple machine learning algorithms. By leveraging the strengths of decision trees, support vector machines, neural networks, and ensemble methods, this approach achieved higher accuracy, sensitivity, and specificity than single-algorithm models. This hybrid framework underscores the importance of integrating diverse techniques to create robust predictive tools for cardiovascular conditions.
*Gart Solutions can help implement similar predictive frameworks by integrating advanced ML models with user-friendly dashboards for clinicians.
Diabetes Classification and Prediction:
A 2020 study examined the classification and prediction of diabetes using machine learning paradigms. It delved into the comparative performance of various ML algorithms, identifying those most effective for diagnosing and assessing diabetes risk. An innovative extension of this work, published in 2022 as “A Novel Diabetes Healthcare Disease Prediction Framework Using Machine Learning Techniques,” introduced a structured framework for diabetes prediction. This research not only improved diagnostic accuracy but also provided a scalable system adaptable to different healthcare settings.
*Gart Solutions’ cloud migration services ensure that healthcare providers can leverage such frameworks without infrastructure constraints.
Comprehensive Disease Risk Analysis:
Another 2020 study on heart disease prediction applied machine learning techniques to evaluate diverse datasets. The models developed in this research highlighted the utility of predictive analytics in pinpointing at-risk individuals even before symptoms arise, paving the way for preventive care strategies.
These case studies collectively illustrate how predictive analytics empowers healthcare providers to transition from reactive care to proactive and personalized medical interventions. By leveraging vast datasets and advanced computational models, healthcare systems can revolutionize how chronic diseases are managed and treated.
Why Choose Gart Solutions?
With expertise in DevOps, cloud migration, and data-driven solutions, Gart Solutions is uniquely positioned to assist healthcare organizations in deploying predictive analytics projects. Our services include:
- Platform Engineering: Tailoring cloud platforms to support predictive models with high scalability and reliability.
- Data Integration: Seamlessly connecting disparate data sources, including EHRs, to enable comprehensive analytics.
- Compliance Assurance: Ensuring solutions adhere to industry standards like HIPAA and GDPR.
Partnering with Gart Solutions means transforming healthcare challenges into opportunities for innovation and better patient care.
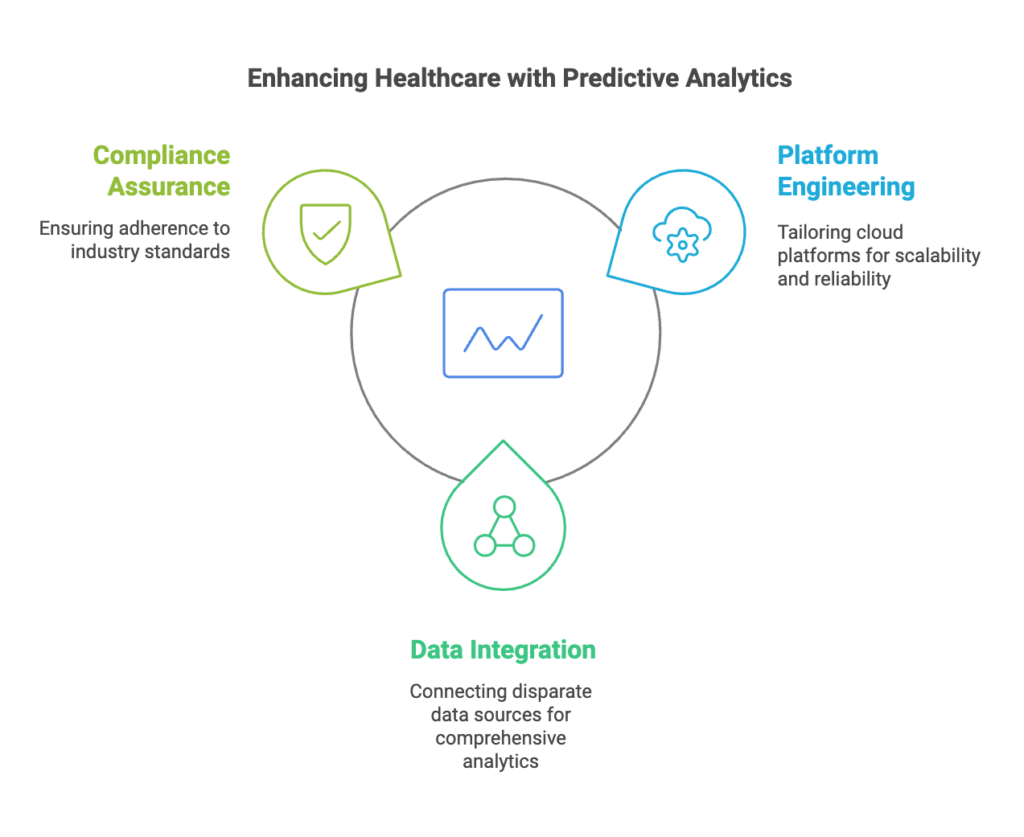
Conclusion
Predictive analytics is revolutionizing healthcare by delivering actionable insights that improve clinical and operational outcomes. The synergy of big data, predictive modeling, and advanced visualization techniques positions healthcare providers to anticipate challenges, deliver superior care, and operate more sustainably. Embracing these technologies enables the industry to address existing inefficiencies while laying the groundwork for a more data-driven future.
See how we can help to overcome your challenges